Probabilistic modeling of open pit resources is well established: simulate at high resolution, average to a selective mining unit (SMU) size that represents future mining operations and grade control information, then report expected resources. This blog addresses probabilistic modeling of underground resources.
The goal of modeling resources is to estimate what could be achieved by future mining activities including the information effect, that is, anticipated additional information. We cannot treat each realization as a truth (because we will never know the deposit exactly) and we cannot treat the high-resolution realizations as an ensemble and optimize over all (because we will get additional production drilling in the future). The correct approach requires consideration of the information effect.
Let me explain this with a small example. Imagine a steeply dipping deposit (black) with some exploration drill holes (red lines). A nominal production volume is shown by the gray square. At the time of exploration there is only one drill hole in the volume, but there are other exploration drill holes and additional drill holes (green) will be available at the time of production. The final stope (light blue) will be designed based on the final data available.
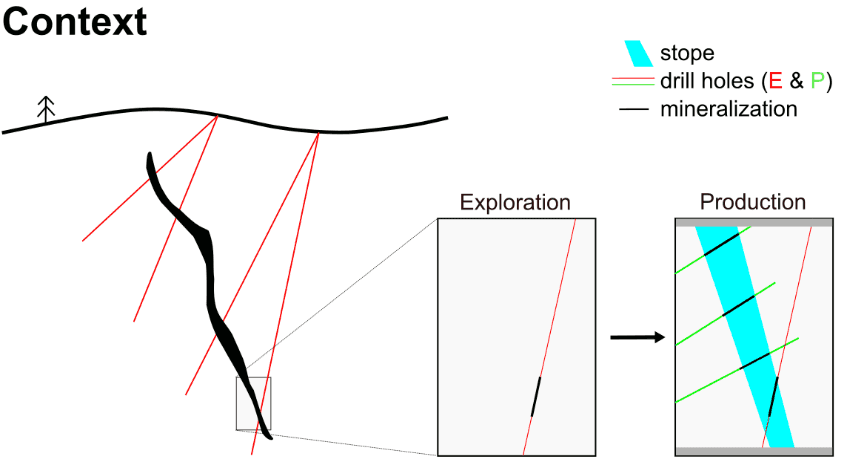
The conventional approach to resource estimation provides a best model based on all available information and the best stope from that model, see below. The stope may be optimistic and overfit. Dilution factors are applied. Geostatistical simulation would assess the uncertainty in the position, volume and quality of the mineralized shape. In these sketches, only the position and volume are shown. The grade would be simulated within the mineralized shape.
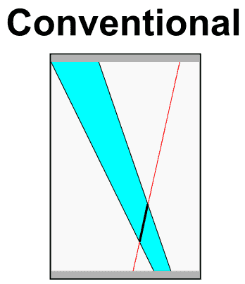
The modern probabilistic approach would create multiple realizations (see below) that represent our uncertainty in the state of nature. The sketch shows three overlapping volumes in shades of green, but we would have 100 to 200 realizations in practice. The simulation would consider surfaces, categorical variables and continuous variables. How do we process these realizations to compute realistic resource estimates?
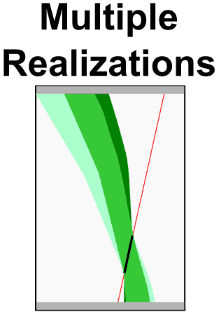
There are two evident naïve approaches: optimize over each realization or optimize over all realizations. Optimizing over each realization assumes perfect knowledge, which is unrealistic. The second alternative is to optimize over all realizations, which is pessimistic – some of the uncertainty will be resolved in the future. In practice, the simulated realizations may have minimal overlap and there may be no stope that provides a net positive value. In general, the risk averse stope would be unreasonably small and the opportunity seeking stope would be unreasonably large. Optimizing over all realizations at the same time would only be appropriate if no new information is coming available. The key challenge with these naïve approaches is that they do not explicitly address the upcoming information before mining.
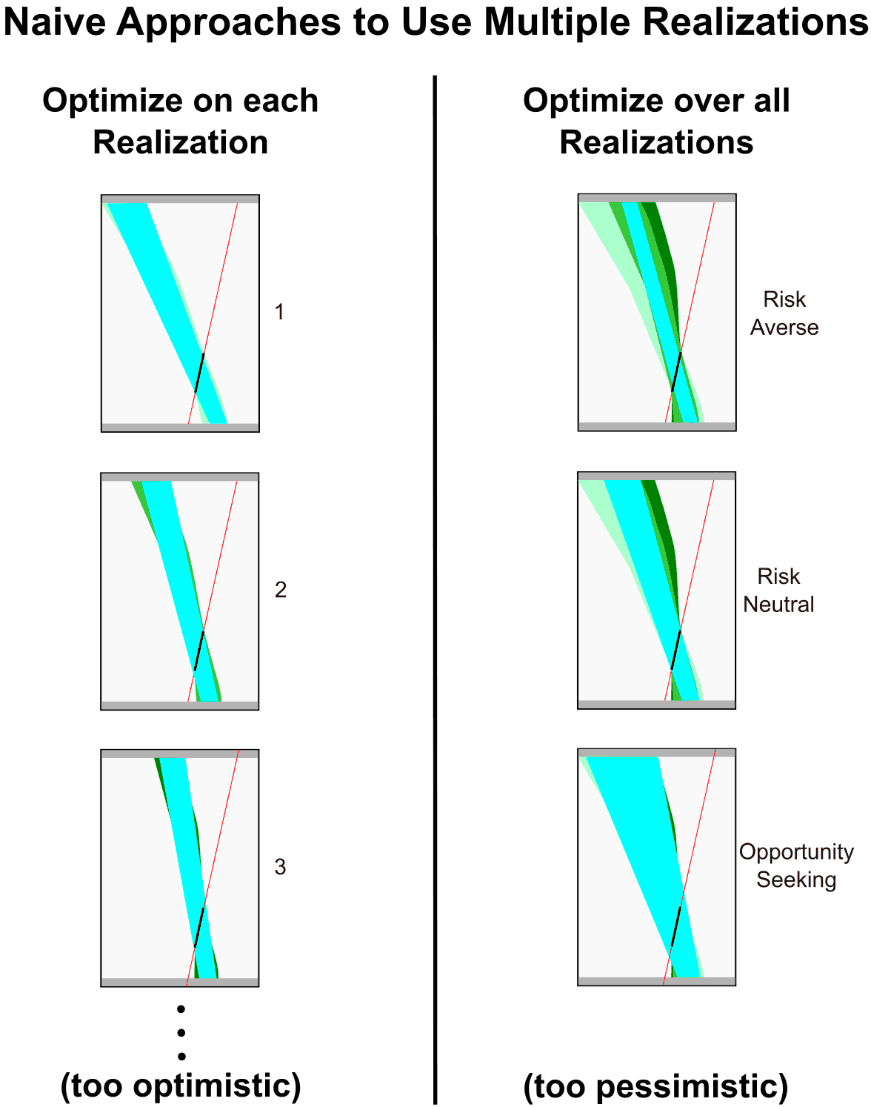
The correct approach requires resampling each realization to consider the variation in the future information. Each realization is sampled, a synthetic grade control model is built, and a stope is designed, see below. The resources (tonnes, grade and quantity of metal) are computed for each realization and a distribution of uncertainty is assembled. The expected resource would be taken after all realizations are processed.
Resource estimation in open pit mining has shortcuts including: (1) the SMU concept containing all aspects of future mining – we do not simulate blast holes (or dedicated grade control drilling), short term modeling and dig limits, (2) localization creating unique SMU models so that planners do not have to accommodate non-uniqueness, and so on. There are no similar shortcuts for underground resources. The only shortcut that may be useful is to apply overly simplistic mineable shapes on each realization as a proxy for resampling and short-term modeling. Optimizing over all realizations simultaneously would only be reasonable if no additional information is coming available.
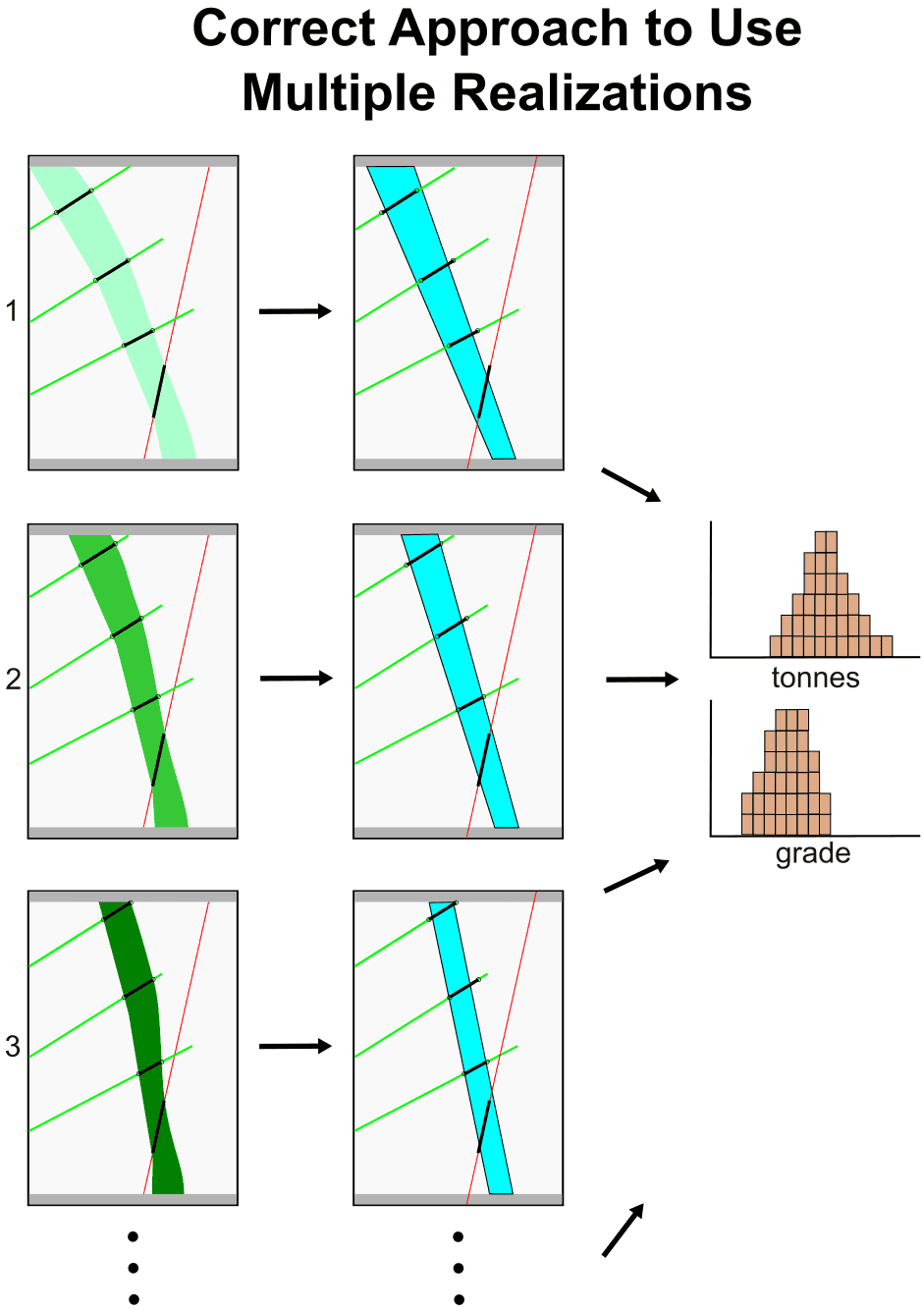
The core principle of simulation is all realizations all the time. The naïve approaches and the correct approach adhere to this principle; however, there are other principles. Namely, simulation should mimic reality (past, present and future) as close as possible. The naïve approaches do not satisfy this – assuming perfect knowledge or no additional information, respectively. The correct approach, however, explicitly considers the information effect.
The correct approach supports risk qualified planning (like SMU uncertainty in an open pit context). These stope resources are analogous to the SMU resources in open pit resources. They must still be sequenced. Optimizing across all realizations is still required; tools analogous to the heuristic pit optimizer from the Centre for Computational Geostatistics (CCG) are applicable.
Resource Modeling Solutions provides the software tools and workflows to estimate probabilistic resources in an efficient and effective manner. Solutions to considering probabilistic resource estimates in mine planning are coming!

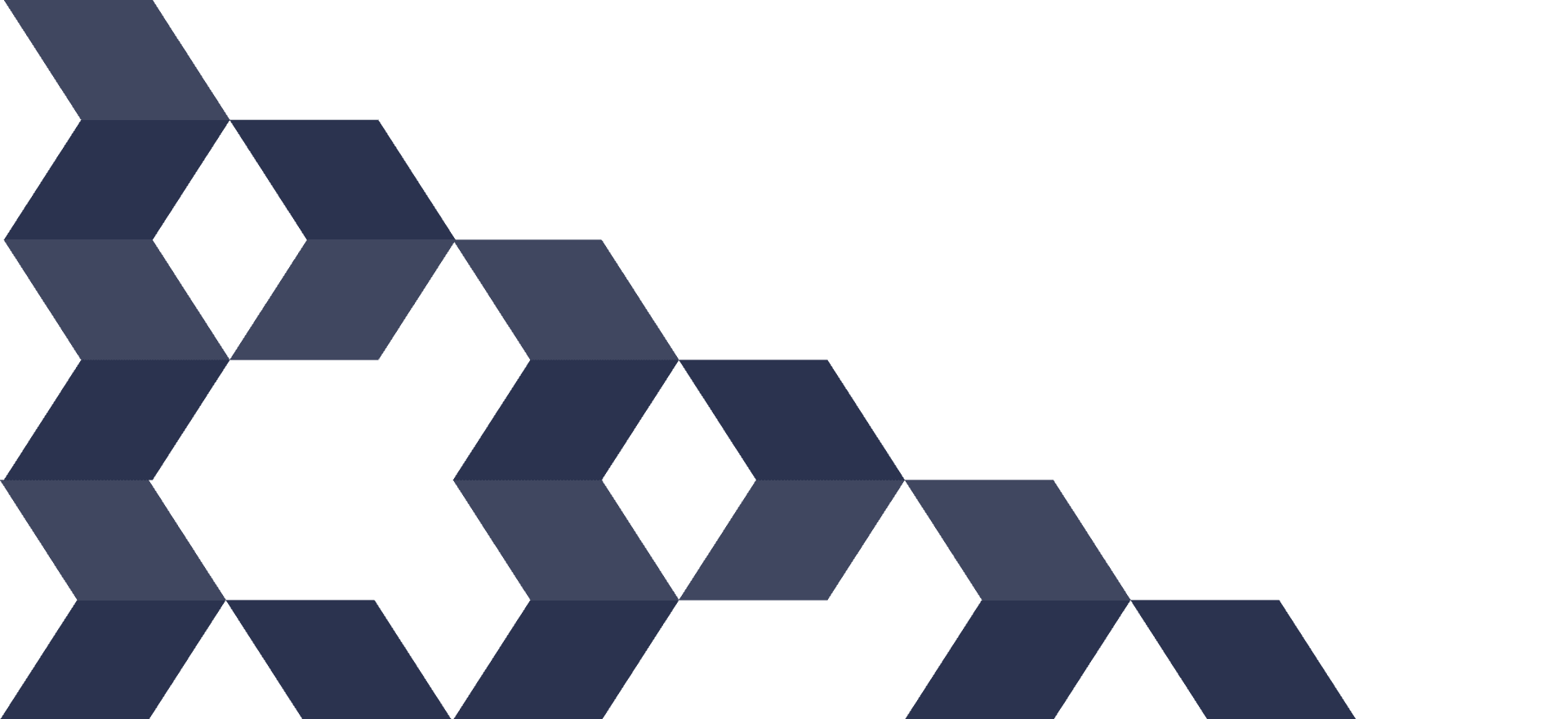