RMSP is relied on by some of the largest mining, petroleum and consulting companies around the globe to effectively characterize their deposits, optimize ore control, and design drill programs that significantly reduce resource uncertainty.
100+
Projects Completed
13
Active Countries
400+%
Project Acceleration Potential
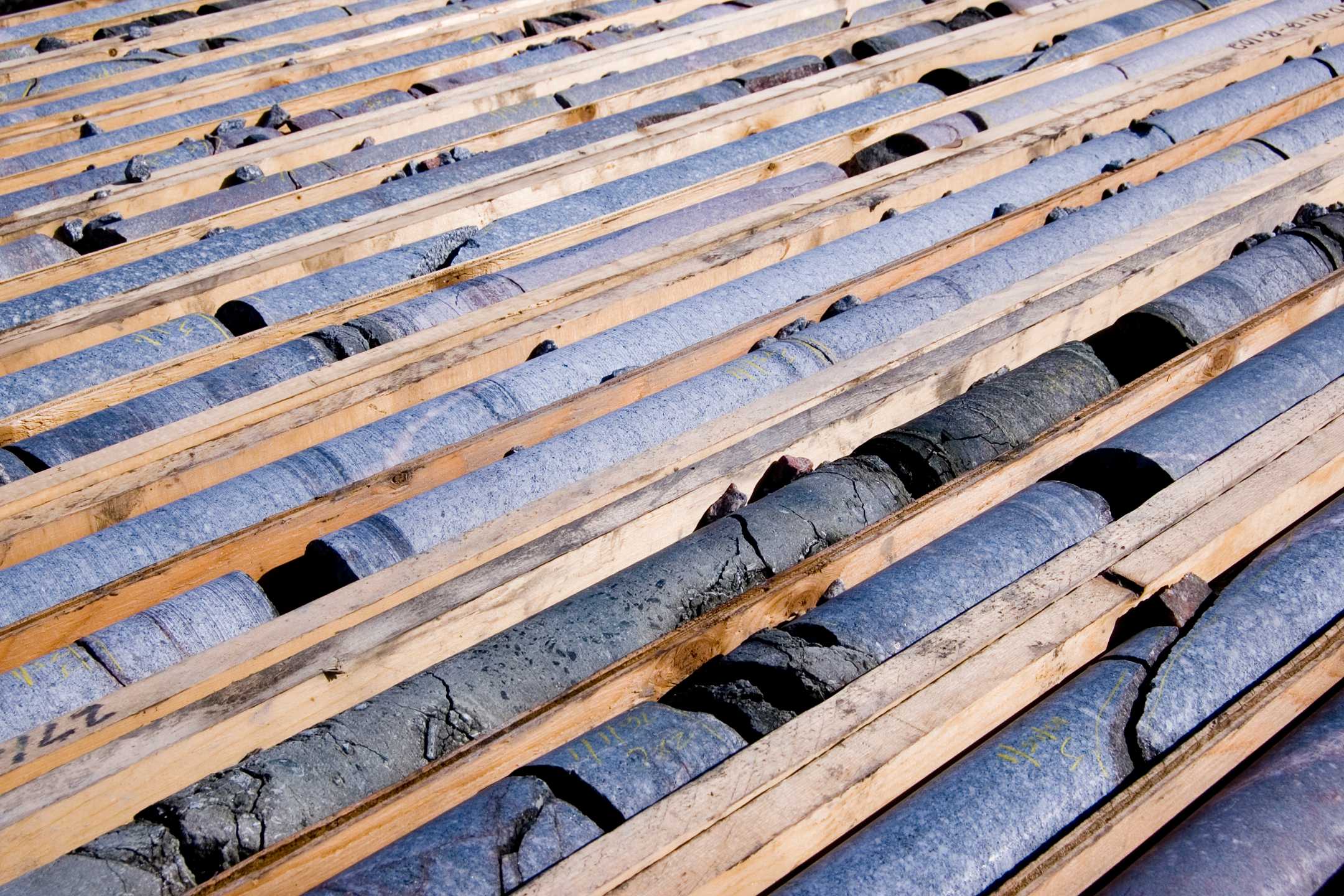
State of the Art Functionality
RMSP integrates hundreds of geostatistical algorithms within the Python ecosystem
Massive Models
Our fully-parallel library supports the largest deposits with hundreds of attributes simultaneously
Seamless Cloud Deployment
Set up a simulation locally then push it to your corporate cloud to crunch hundreds of realizations
Consultant Support
Backed by our team of consultants who help with customizing and auditing your workflows
How We Differ From Our Competitors
Comprehensive geostatistical tools that combine fundamental and proven state-of-the-art techniques
Designed from the ground-up to be optimized across modern parallel processing and cloud deployment
Supported by a team of Geostatisticians that blend academic and industry expertise for development and training
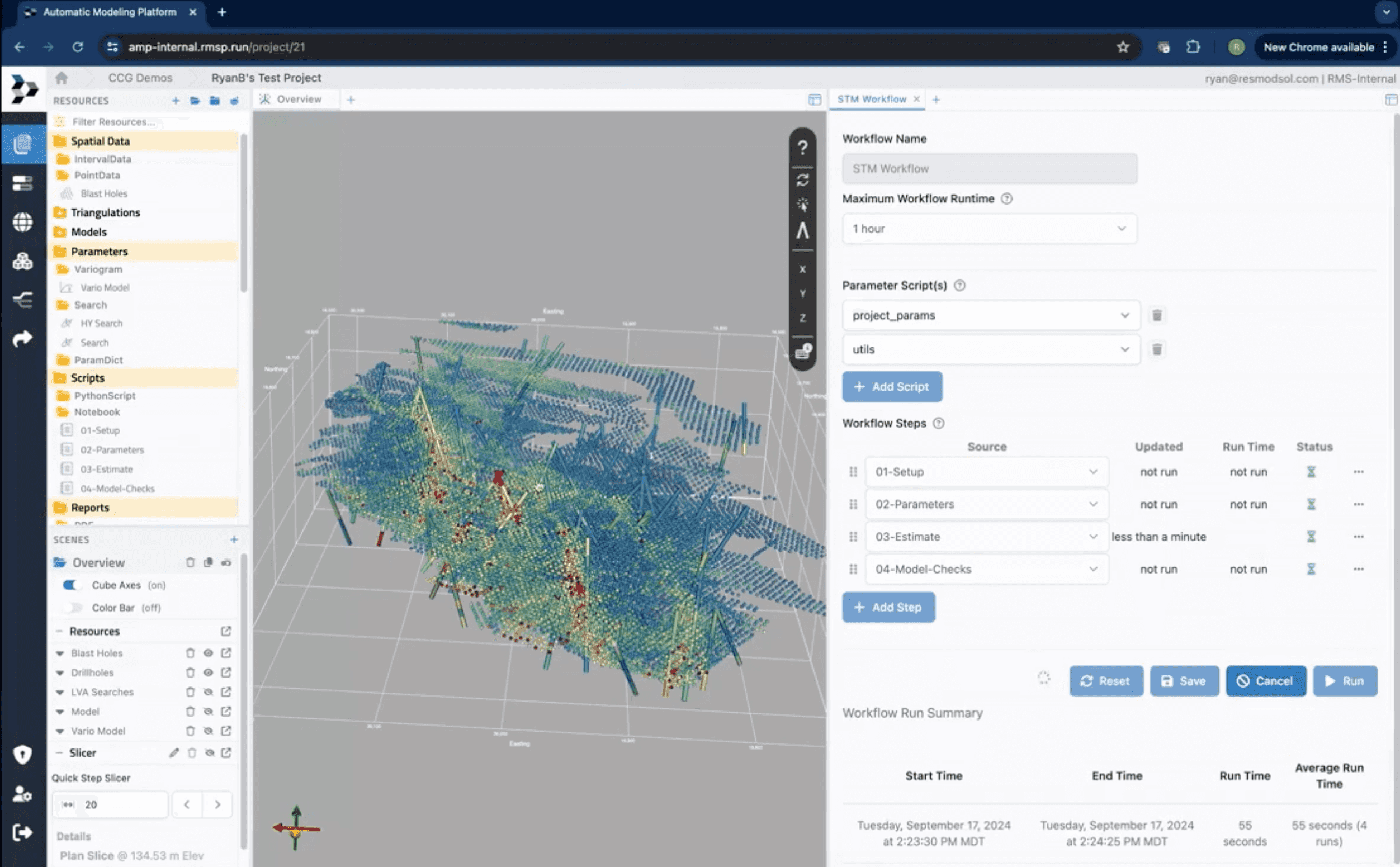
View Full Example
Designed by Geostatisticians for Geostatisticians
RMSP gives you the right tools to solve your geostatistical modeling challenges
With over 100 geostatistical modeling functions, including variogram calculation and modeling, estimation with kriging, uniform conditioning, projection pursuit multivariate transform simulations and more, RMSP is the most advanced geostatistical modeling software available today.
Is RMSP Right For Your Next Project?
Our team of highly qualified geostatisticians provide one-on-one support, training programs, and continued software development so you can integrate RMSP into your job site seamlessly.
Thinking of Getting RMSP Training?
Need more information? Book a training session with one of our industry experts to explore the endless capabilities of RMPS and how it can be leveraged for your specific project.